The 14th International Workshop on Genetic Improvement @ICSE 2025

Navigation: Attending, Important Dates, Keynote, CFP, Workshops Chairs, Program Committee
Attending
The 14th instalment of the GI workshop is will take place in Ottawa, collocated with the 47th International Conference on Software Engineering, ICSE 2025, which will be held in Ottawa’s Shaw Centre.
The Shaw Centre is part of a large building complex that also includes the Rideau Centre (a shopping mall), the Westin Hotel, and the underground Rideau light rail station. It overlooks the Rideau Canal, a UNESCO World Heritage site, and is a short walk from the Byward market, Parliament, the National Arts Centre (performing arts), the National Gallery of Canada, and the University of Ottawa. It is also a short walk from most of the other hotels that have been booked for the ICSE conference.
Click here for information about how to get to the Shaw Centre, and click here for information about how to get to Ottawa, as well as visa and travel authorization information.
Click here for information about registration.
The workshop is expected to be held in-person. In case of a virtual or hybrid event, virtual presentations may be possible.
Important Dates
Submission Deadline: 11 November 2024 (Mon)Extended Submission Deadline: 14 November (Thurs)Notification: 8 December 2024 (Sun)Camera-ready: 5 February 2025 (Wed)- Workshop: 27 April 2025 (Sun)
Keep up to date with the latest event news via our Twitter: https://twitter.com/gi_of_software.
Keynote
We are happy to announce that Dr. Shin Hwei Tan, head of the Automated Program RepaIr and MaintEnance Lab (A-PRIME), Concordia University Montreal, Canada, will give the keynote speech at GI@ICSE 2025.
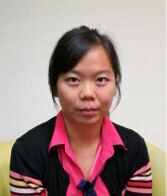
Put on Your Tester Hat: Improving programs for Automated Program Generation
Given either a specification written in natural language or an input program, automated program generation techniques produce a program according to the given specification or by modifying the input program. Automated program generation is a powerful technique that can be used for finding bugs in software systems that take programs as input or fixing bugs in the input programs. However, most existing techniques focus on automated program generation for automated program repair or program synthesis. This talk introduces a different perspective of automated program generation where we will discuss our latest results on automated program generation for testing static program analyzers by designing different types of program transformations. We will also explore and rethink about the automated program generation problem from the tester perspective. The new perspective could have huge potential for the design of new genetic improvement techniques to improve programs for automated program generation.
Shin Hwei Tan is an Associate Professor (Gina Cody Research Chair) in Concordia University. Before moving to Concordia University, she was an Assistant Professor in Southern University of Science and Technology in Shenzhen, China. She obtained her PhD degree from National University of Singapore and her B.S (Hons) and MSc degree from University of Illinois at Urbana-Champaign. Her main research interests are in automated program repair, software testing and open-source development. She is an Associate Editor for TOSEM and the Guest Editors-in-Chief for the New Frontier in Software Engineering track in TOSEM. She has also served as PCs for top-tier software engineering conferences, where she won 3 best reviewers award (FSE 2020, ASE 2020, ICSE 2022 NIER-track). She is also the general chair of FSE26 which will be held in Concordia University.
Accepted Papers
A Three-Stage Genetic Algorithm for Compiler Flag and Library Version Selection to Minimize Execution Time
by Chi Ho Chan and Spyro Nita
PDF VIDEO URL
Abstract
Automated Software Performance Improvement with Magpie
by Aymeric Blot
PDF SLIDES URL
Abstract
Empirical Comparison of Runtime Improvement Approaches: Genetic Improvement, Parameter Tuning, and Their Combination
by Thanatad Songpetchmongkol, Aymeric Blot, and Justyna Petke
PDF PDF VIDEO URL
Abstract
Enhancing Software Runtime with Reinforcement Learning-Driven Mutation Operator Selection in Genetic Improvement
by Damien Bose, Carol Hanna, and Justyna Petke
PDF VIDEO URL URL
Abstract
LLM-Assisted Crossover in Genetic Improvement of Software
by Dimitrios Stamatios Bouras, Justyna Petke, and Sergey Mechtaev
PDF VIDEO URL
Abstract
Large Language Model based Code Completion is an Effective Genetic Improvement Mutation
by Jingyuan Wang, Carol Hanna, and Justyna Petke
PDF VIDEO URL URL
Abstract
Put on Your Tester Hat: Improving programs for Automated Program Generation
by Shin Hwei Tan
PDF
Abstract
The gem5 C++ glibc Heap Fitness Landscape
by William B. Langdon and Bobby R. Bruce
PDF VIDEO URL
Abstract
Schedule
This schedule appears in Ottawa’s time zone (GMT-5, Greenwich Mean Time, summer); compare to your timezone here.
Presentations for full papers are 20 minutes long, followed by 10 minutes for questions.
Presentations for short papers consist of a 10 minute talk, followed by 5 minutes for questions.
The workshop will take place in Roger Centre.
See also the schedule on the ICSE website for easy bookmarking.
- Sunday 27 April 2025 09:00-10:30 (90 minutes; chairs: Aymeric Blot and Justyna Petke)
- Sunday 27 April 2025 11:00-12:30 (90 minutes; chairs: W.B. Langdon and Penn Faulkner Rainford)
- 11:00: Large Language Model based Code Completion is an Effective Genetic Improvement Mutation — Jingyuan Wang, Carol Hanna, and Justyna Petke (20+10 minutes)
- 11:30: Enhancing Software Runtime with Reinforcement Learning-Driven Mutation Operator Selection in Genetic Improvement — Damien Bose, Carol Hanna, and Justyna Petke (20+10 minutes)
- 12:00: Empirical Comparison of Runtime Improvement Approaches: Genetic Improvement, Parameter Tuning, and Their Combination — Thanatad Songpetchmongkol, Aymeric Blot, and Justyna Petke (20+10 minutes)
- 12:30: Lunch (90 minutes)
- Sunday 27 April 2025 14:00-15:30 (90 minutes; chairs: Carol Hanna and Vesna Nowack)
- 14:00: Invited Tutorial — Automated Software Performance Improvement with Magpie — Aymeric Blot (5+55+15 minutes Q&A)
- 15:15: A Three-Stage Genetic Algorithm for Compiler Flag and Library Version Selection to Minimize Execution Time — Chi Ho Chan and Spyro Nita (10+5 minutes)
- 15:30: Break & Afternoon tea (30 minutes)
- Sunday 27 April 2025 16:00-17:30 (90 minutes; chairs: Thanatad Songpetchmongkol and Oliver Krauss)
- 16:00: The gem5 C++ glibc Heap Fitness Landscape — William B. Langdon and Bobby R. Bruce (20+10 minutes)
- 16:30: LLM-Assisted Crossover in Genetic Improvement of Software — Dimitrios Stamatios Bouras, Justyna Petke, and Sergey Mechtaev (20+10 minutes)
- 17:00: Discussion (25 minutes; chairs: Aymeric Blot and Oliver Krauss)
- 17:25: Awards and Closing (5 minutes; chair: Aymeric Blot & presenter: Dr Shin Hwei Tan)
Call For Submissions - Submit Here
We invite submissions that discuss recent developments in all areas of research on, and applications of, Genetic Improvement. The International Workshop on Genetic Improvement is the premier workshop in the field and provides an opportunity for researchers interested in automated program repair and software optimisation to disseminate their work, exchange ideas, and discover new research directions.
Topics of interest include both the theory and practice of Genetic Improvement. Applications of GI include, but are not limited to, using GI to:
- Improve runtime efficiency
- Decrease memory consumption
- Decrease energy consumption
- Transplant new functionality
- Specialise software
- Generate multiple versions of software
- Improve low level or binary code
- Use of Large Language Models with GI
- Use of AI with GI
- Repair bugs
- GI techniques in industrial settings
The workshop emphasises interaction and discussion between participants.
Authors are encouraged to submit early and in-progress work.
We invite two types of submissions:
- Research papers (eight page limit, including references)
- Position papers (two page limit, including references)
Papers should be submitted electronically here and must conform to the IEEE conference proceedings template as per the ICSE submission process (template)
Accepted papers must be presented at GI 2025 and will appear in the ICSE workshops volume. The official publication date of the workshop proceedings is the date the proceedings are made available by IEEE. This date may be up to two weeks prior to the first day of ICSE 2025. The official publication date affects the deadline for any patent filings related to published work.
The best paper and best presentation will be awarded during the workshop.
Following the workshop selected papers will be invited to submit to a special issue of the Automated Software Engineering journal on “Genetic Improvement: Pushing the Boundaries of Automated Software Development”
Workshop Chairs
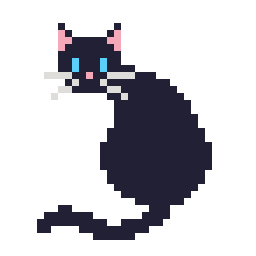
Aymeric Blot is a Senior Lecturer at the University of Rennes and a member of the IRISA research centre in the joint Inria/IRISA DiverSE team. After receiving a doctorate from the University of Lille, focused on automated algorithm design for multi-objective combinatorial optimisation, they moved to University College London to work on software specialization using genetic improvement. Currently working on developing and maintaining the Magpie automated software improvement framework.
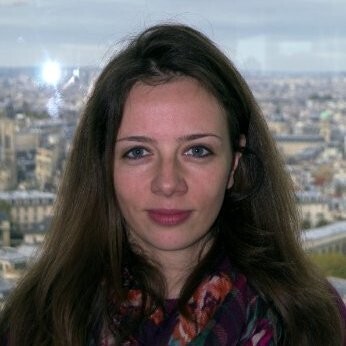
Vesna Nowack received her PhD in Computer Architecture at UPC, Spain (2016). Recently, she worked on APR in academia (Queen Mary University of London, Lancaster University) and industry (Bloomberg). She is currently a Research Associate at Imperial College London with a focus on human-in-the-loop ML systems.
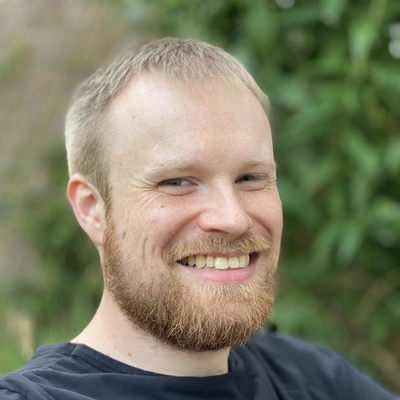
Oliver Krauss received his doctorate in 2022 in Pattern Mining and Genetic Improvement in Compilers and Interpeters. His research focuses on mining patterns in software, as well as data, to improve runtime performance and energy consumption. He maintains several open source frameworks, such as Amaru.
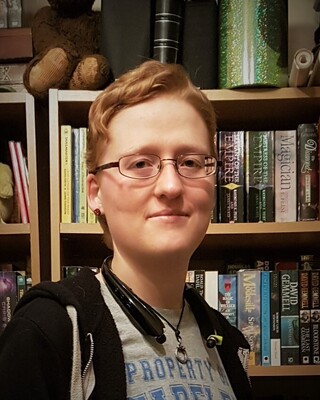
Penn Faulkner Rainford received their PhD in 2019 from the University of York. They work on the interface of Biochemistry and Computing (University of York). Their GI research centres on applications of principles of natural evolution to genetic improvement. Currently focused on phylogenetic analysis for systems designed for real-time evolutionary adaptation (Lancaster University).
Program Committee
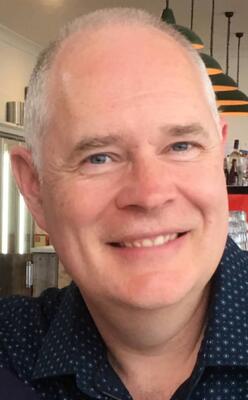
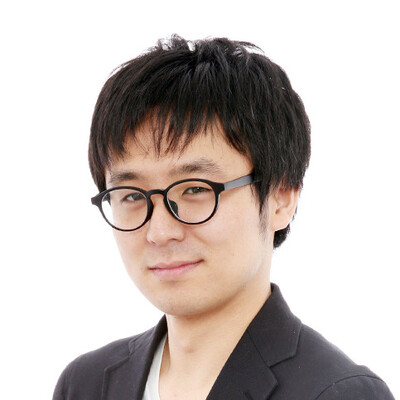
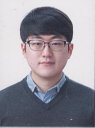
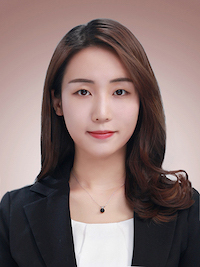
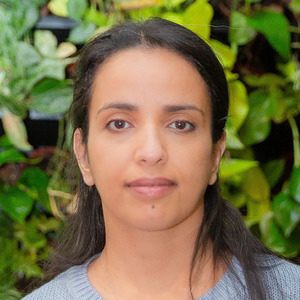
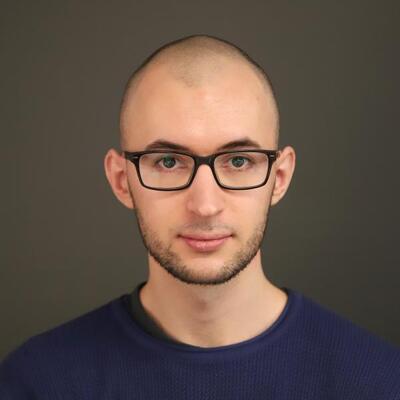
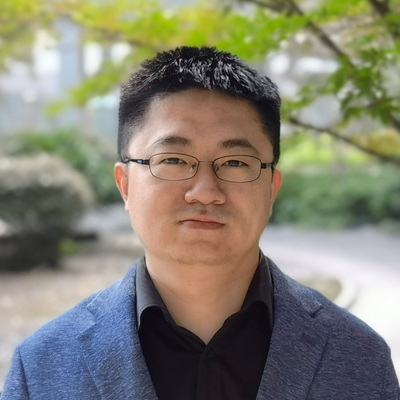
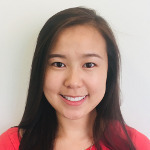
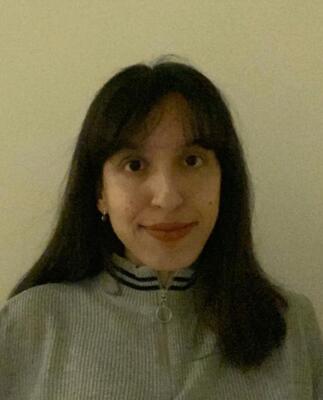
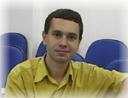
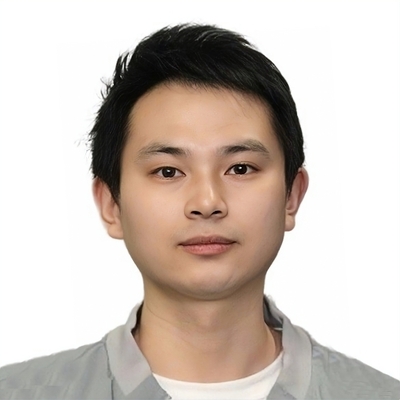
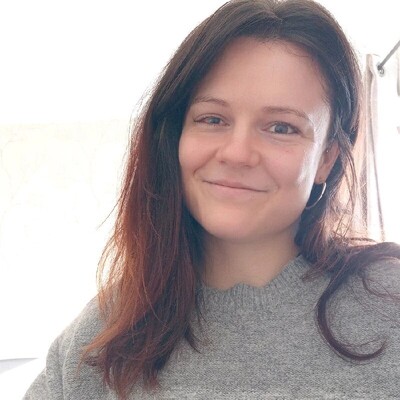
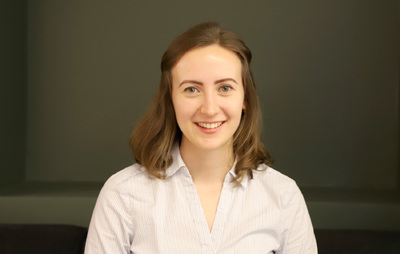
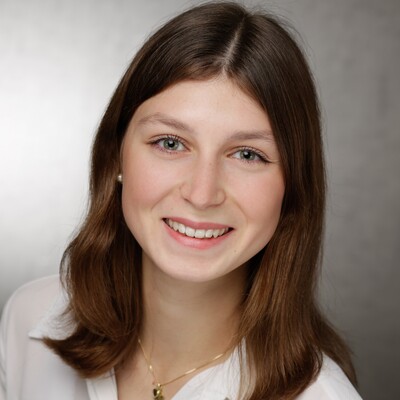
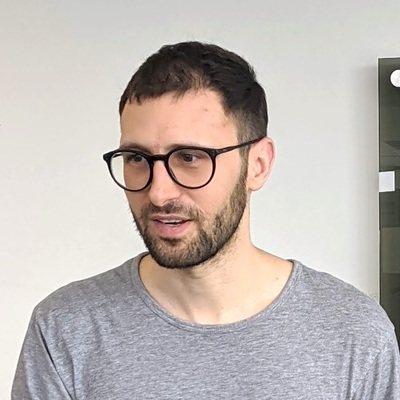
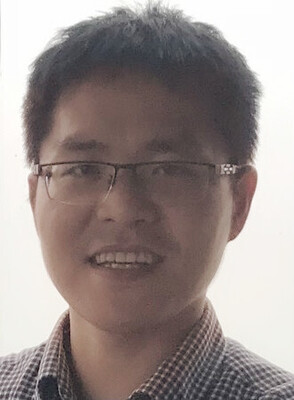
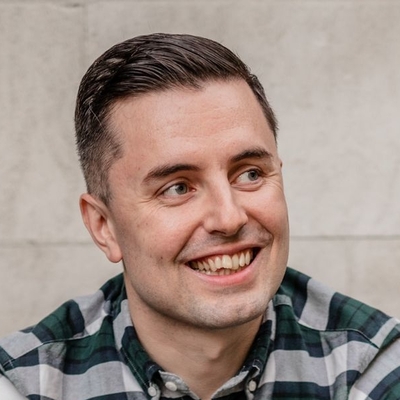
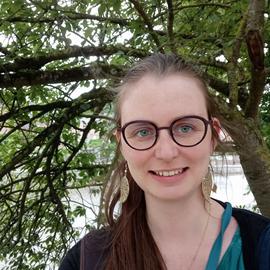
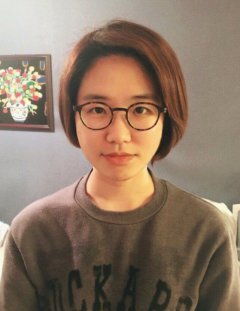
ICSE 2025 Sponsors